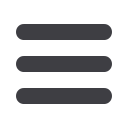
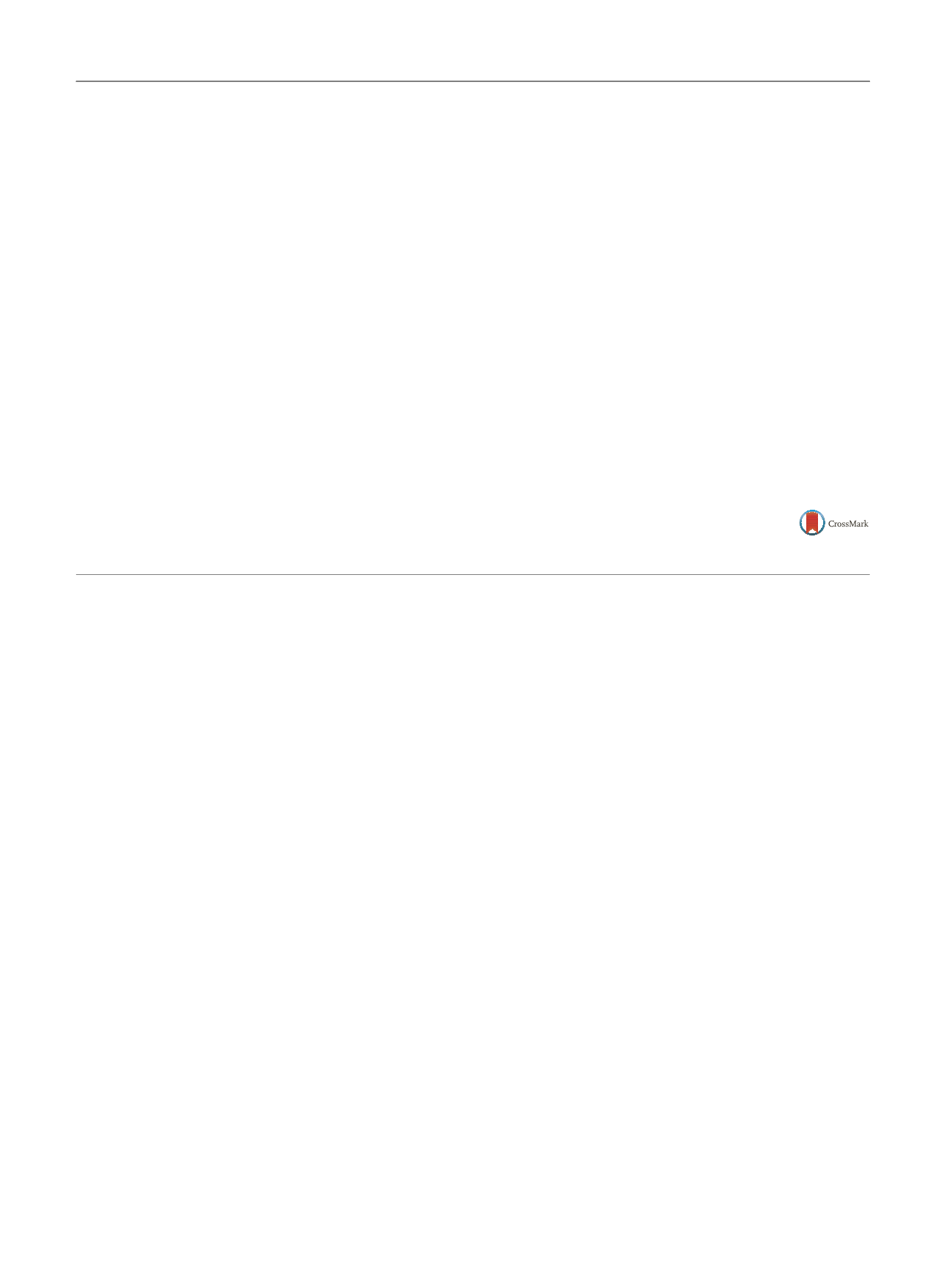
Finland, Juvenile Diabetes Research Foundation, and Sigrid Juselius
Foundation. The authors thank Pirjo Haavisto, Taina Lahti, and Katri Villa
from Turku University Hospital for assistance in the manual data
collection, and Aidan McGlinchey for checking the English language.
Appendix A. Supplementary data
Supplementary material related to this article can be
found, in the online version, at
http://dx.doi.org/10.1016/j. eururo.2017.01.043 .References
[1]
Guinney J, Wang T, Laajala TD, et al. Prediction of overall survival for patients with metastatic castration-resistant prostate cancer: de- velopment of a prognostic model through a crowdsourced chal- lenge with open clinical trial data. Lancet Oncol 2017;18:132–42.[2]
Sydes MR, Langley RE. Potential pitfalls in the design and reporting of clinical trials. Lancet Oncol 2010;11:694–700.[3]
Templeton AJ, Vera-Badillo FE, Wang L, et al. Translating clinical trials to clinical practice: outcomes of men with metastatic castra- tion resistant prostate cancer treated with docetaxel and predni- sone in and out of clinical trials. Ann Oncol 2013;24:2972–7.[4]
Howard DN, Chambers C, Cusano F. Efficacy vs. effectiveness— docetaxel and prednisone in hormone refractory prostate cancer. J Oncol Pharm Pract 2008;14:45–9.[5]
Halabi S, Lin C-Y, Kelly WK, et al. Updated prognostic model for predicting overall survival in first-line chemotherapy for patients with metastatic castration-resistant prostate cancer. J Clin Oncol 2014;32:671–7.a
Turku Centre for Biotechnology, Turku, Finland
b
Department of Mathematics and Statistics, University of Turku, Turku,
Finland
c
Department of Information Technology, University of Turku, Turku, Finland
d
Department of Oncology and Radiotherapy, Turku University Central
Hospital, Turku, Finland
e
Department of Clinical Oncology, University of Turku, Turku, Finland
f
Department of Pulmonary Diseases and Clinical Allergology, Turku
University Hospital and University of Turku, Turku, Finland
g
Centre for Clinical Informatics, Turku University Hospital, Turku, Finland
*Corresponding author. Computational Biomedicine and Bioinformatics,
Turku Centre for Biotechnology, Tykisto¨katu 6, FI-20520 Turku, Finland.
Tel. +358 2 333
[1_TD$DIFF]
8009; Fax: +358 2
[2_TD$DIFF]
215
[3_TD$DIFF]
8808.
E-mail address:
laura.elo@utu.fi(L.L. Elo).
January 24, 2017
http://dx.doi.org/10.1016/j.eururo.2017.01.043Methodological Considerations for Early-phase Development of
Immune Checkpoint Inhibitors in Urothelial Bladder Cancer
Andrea Necchi
* ,Daniele Giardiello, Luigi Mariani
Early results from immunotherapy trials paved the way for
a revolutionary approach in urothelial carcinoma (UC).
However, the published single-arm studies on atezolizu-
mab and durvalumab, added to other published studies,
raised important hints on interpretation of results accord-
ing to their study design in a context involving substantial
uncertainties regarding PD-L1 immunohistochemical (IHC)
expression
[1,2]. In general, should any similar investigation
suggest that the target treatment benefit is more likely to
occur in a specific biomarker-defined subset of patients, any
further study might be more suitably decided in accordance
with an enrichment strategy. Consolidated statistical
criteria for supporting such a decision are lacking in the
context of noncomparative trials with binary endpoints,
such as those commonly adopted in phase 2 trials in
oncology. One solution with which we have had satisfactory
experience is based on the Bayesian approach of predictive
probability (PP) calculation.
As explained by Lee and Liu
[3], PP quantifies the
probability of reaching a positive result by the end of the
trial on the basis of cumulative information in the current
stage. Therefore, PP can be used to decide on early stopping
because of efficacy/futility or to continue the study when
the current data are not yet conclusive. Our approach to
biomarker-stratified studies is to calculate PP both for the
overall sample and separately for each stratum, and to
allow study continuation only when PP
>
30% (in which
case we denote the treatment as promising). Enrichment in
particular is considered when PP in the best-performing
stratum fulfills the 30% criterion and exceeds by
>
20% the
estimate for the competing stratum. At the same time,
enrichment is assessed in terms of the projected time for
study completion (biomarker-driven patient selection
implies a reduction in the accrual rate, which can be more
severe in the case of low feature prevalence) and finally
adopted if not too much penalizing. The PP approach can
also be used from scratch for sequential study planning
[3],
but this choice would not have the flexibility necessarily
required in biomarker-stratified studies.
As an example, we applied our approach to the
durvalumab data
[1] ,hypothesizing an overall response rate
(ORR) of
>
20% as the efficacy criterion and a maximum
E U R O P E A N U R O L O G Y 7 1 ( 2 0 1 7 ) 8 3 7 – 8 4 3
840