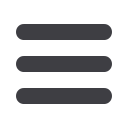
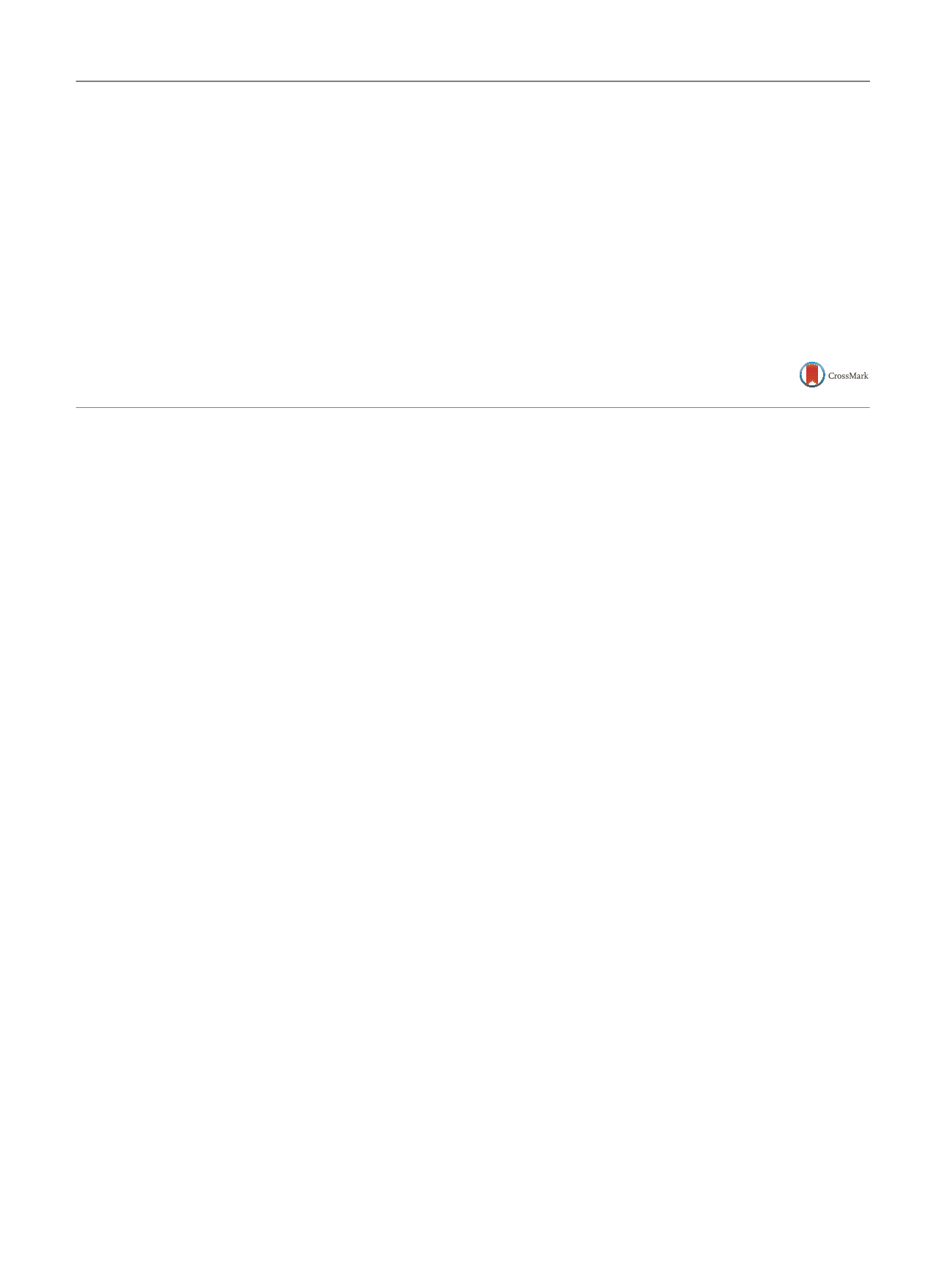
Conflicts of interest:
The authors have nothing to disclose.
References
[1]
Lopes Neto AC, Korkes F, S ilva 2nd JL, et al. Prospective randomized study of treatment of large proximal ureteral stones: extracorporeal shock wave lithotripsy versus ureterolithotripsy versus laparosco- py. J Urol 2012;187:164–8.[2]
Mugiya S, Ozono S, Nagata M, Takayama T, Nagae H. Retrograde endoscopic management of ureteral stones more than 2 cm in size. Urology 2006;67:1164–8.[3]
Moufid K, Adermouch L, Amine M, Lezrek M, Touiti D, Abbaka N. Large impacted upper ureteral calculi: a comparative study be- tween retrograde ureterolithotripsy and percutaneous antegrade ureterolithotripsy in the modified lateral position. Urol Ann 2013;5:140.[4]
Scoffone CM, Cracco CM, Cossu M, Grande S, Poggio M, Scarpa RM. Endoscopic combined intrarenal surgery in Galdakao-modified supine Valdivia position: a new standard for percutaneous nephro- lithotomy? Eur Urol 2008;54:1393–403.Urology Department, Kaohsiung Medical University Hospital,
Kaohsiung, Taiwan
*Corresponding author. Urology Department, Kaohsiung Medical
University Hospital, 100 Tzyou 1st Road, Kaohsiung 807, Taiwan.
Tel. +88 609 28689935.
E-mail address:
sculptor39@yahoo.com.tw(T.-Y. Huang).
October 11, 2016
http://dx.doi.org/10.1016/j.eururo.2016.10.019How Reliable are Trial-based Prognostic Models in Real-world
Patients with Metastatic Castration-resistant Prostate Cancer?
Fatemeh Seyednasrollah
a , b, Mehrad Mahmoudian
a , c, Liisa Rautakorpi
d
,
Outi Hirvonen
d
,
e
, Tarja Laitinen
f
,
g
, Sirkku Jyrkkio¨
d
, Laura L. Elo
a , *Robust prognostic factors are crucial for improving clinical
trial design and later assisting treatment decision-making.
The Dialogue for Reverse Engineering Assessments and
Methods committee recently organized a crowdsourced,
international competition to develop a new prognostic
benchmark for predicting overall survival (OS) of metastatic
castration-resistant prostate cancer (mCRPC) patients in
docetaxel arms of randomized controlled trials (RCTs)
[1] .However, utility of these trial-tailored prognostic
models lacks confirmation in everyday practice.
RCTs are
gold standard
for efficacy assessment of cancer
therapies
[2] ,but agreement of results between RCTs and
real-world (RW) patients remains controversial. RCTs have
high internal, but limited external, validity
as RCT
participants may poorly represent the RW population
[3].
Inspired by promising results from the Dialogue for
Reverse Engineering Assessments and Methods Challenge,
we investigated both consistency between RCT and RW
patients, and the applicability of RCT-based models to RW
patients. The RCT data included four independent phase
3 clinical trials from the Challenge (
n
= 2070). The RW data
included all mCRPC patients (
n
= 289) treated with first-line
docetaxel at Turku University Hospital, Finland, in 2004–
2015 (Supplementary data). Over 150 clinical variables
were available (Supplementary Table 1).
As previously reported
[3,4] ,RW patients tended to be
older and had worse Eastern Cooperative Oncology Group
status than RCT patients (
p
<
0.001; Supplementary
Table 2). However, principal component analysis suggested
high similarity between the cohorts in terms of variables of
the Challenge reference model, Halabi et al.
[5]
(
Fig. 1
A),
supported by consistent hazard ratios for OS across cohorts
(Supplementary Table 3). Contrary to previous studies, OS
was not significantly different between the cohorts
(
p
= 0.11; Supplementary Fig. 1).
Having confirmed similarity between RW and RCT
cohorts, we studied applicability of the three best-
performing (Team 1–3) models from the Challenge and
formerly-developed Halabi reference model to predict OS
of RW mCRPC patients. Although overall model perfor-
mance was lower in RW data than in the Challenge
validation cohort (integrated area under curve 0.724–
0.731 vs 0.743–0.792; Supplementary Table 4), it was
more stable towards the end of follow-up (
Figs. 1B and
1C
). Notably, the Team 1 model outperformed all other
models in the Challenge, but here in RW patients, all
models performed similarly (Bayes factor
<
3). Model
calibration confirmed that the observed survival propor-
tions were in line with predicted survival risk scores
(Supplementary Fig. 2). With equally-performing models,
those with fewer features are potentially more practical.
Team 2, Team 3, and the Halabi model involved eight to
22 features, compared with the Team 1 model with over
90 features and their interactions (Supplementary Table 4,
Supplementary Fig. 3).
Finally, among RW patients fulfilling RCT eligibility
criteria (
n
= 245, Supplementary data, Supplementary
Table 5), the Team 2 model performed best (integrated
area under curve = 0.739 vs 0.701–0.721, Bayes factor
>
3;
Supplementary Table 4). After 24 mo, all models performed
E U R O P E A N U R O L O G Y 7 1 ( 2 0 1 7 ) 8 3 7 – 8 4 3
838